Python has become the leading programming language for computer science and data science, offering unparalleled ease of use and a vast library ecosystem. Its versatility extends from basic scripting to advanced applications such as machine learning and natural language processing (NLP).
This article introduces Python’s key concepts, focusing on control structures, data structures, advanced algorithms, and specialized applications.
Control Statements and Program Development
Python’s control statements play a critical role in directing the flow of a program. These constructs allow developers to design dynamic and efficient code. Below is a closer look at the fundamental control structures in Python:
1. Conditional Statements
Conditional statements enable decision-making by executing code based on specific conditions. The if, elif, and else constructs check logical expressions and execute corresponding blocks of code. This allows developers to create programs that adapt to different inputs or situations.
Example:
age = 20
if age >= 18:
print("Eligible to vote")
else:
print("Not eligible")
Here, the program evaluates whether age is greater than or equal to 18 and runs the appropriate block of code. Conditional statements are essential in scenarios like authentication systems, decision trees, and user input validation.
2. Loops
Loops like for and while allow repetitive execution of a block of code. A for loop iterates over sequences like lists, strings, or ranges, while a while loop runs as long as a condition holds true.
Example:
for i in range(5):
print(f"Iteration {i}")
Loops are particularly useful for processing datasets, automating repetitive tasks, and implementing algorithms like searching and sorting.
3. Break and Continue
break is used to exit a loop prematurely, while continue skips the current iteration and moves to the next. These statements provide granular control over loop execution.
Example:
for i in range(5):
if i == 3:
break # Exits the loop
if i == 1:
continue # Skips the rest of the iteration
print(i)
Control statements are the building blocks of program development, enabling the creation of dynamic, interactive, and efficient applications.
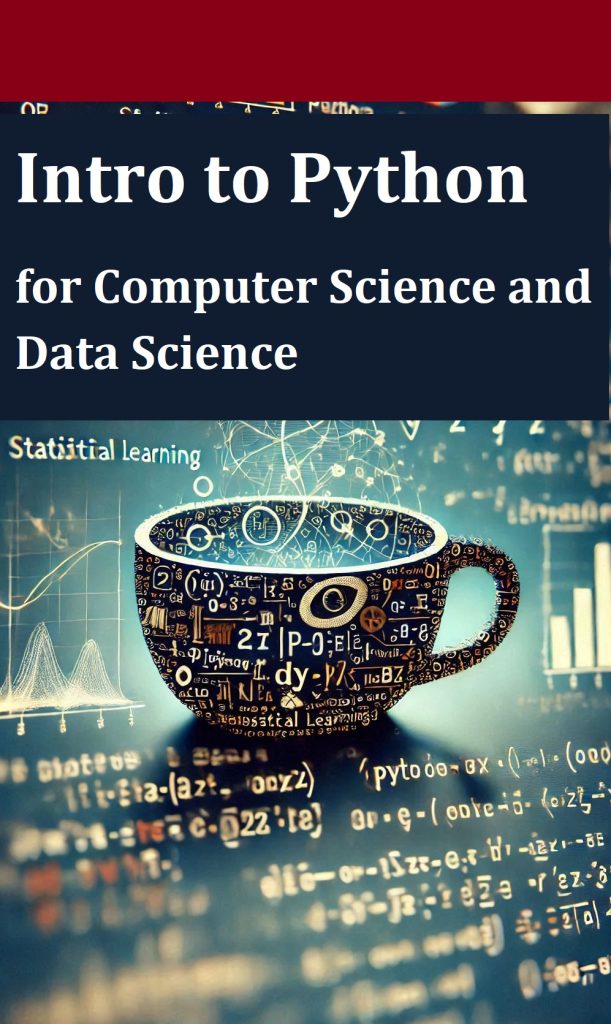
DOWNLOAD PDF: Intro to Python for Computer Science and Data Science
Sequences: Lists and Tuples
Python sequences are essential data structures used to store collections of data. They provide flexibility for organizing and manipulating data in various formats. Two common sequence types in Python are lists and tuples, each with unique characteristics and use cases.
1. Lists
Lists are mutable, meaning their content can be modified after creation. They are ordered, allowing elements to be accessed using their index positions. Lists are ideal for tasks where the data collection needs frequent updates, such as appending, removing, or altering elements.
For example:
fruits = ["apple", "banana", "cherry"]
fruits.append("orange") # Adds "orange" to the list
print(fruits) # Output: ['apple', 'banana', 'cherry', 'orange']
Lists can hold elements of different types, including nested lists, making them versatile. They are used for dynamic data storage, such as managing user input, storing results, or maintaining dynamic datasets in programs.
2. Tuples
Tuples are immutable, meaning their elements cannot be changed once assigned. This immutability makes tuples suitable for storing fixed, constant data that should remain unchanged throughout the program. Tuples are also ordered, and their elements can be accessed using indices.
For example:
coordinates = (10, 20)
print(coordinates[0]) # Output: 10
Tuples are often used in situations requiring integrity and safety, such as representing database keys, function returns, or fixed configurations. Their immutability ensures the data remains secure from accidental modifications.
Object-Oriented Programming in Python
Object-Oriented Programming (OOP) is a paradigm in Python that allows developers to organize and manage code using objects, which represent real-world entities. By using classes and objects, OOP promotes modular, reusable, and maintainable code. Key principles of OOP include encapsulation, inheritance, polymorphism, and abstraction, all of which enhance Python’s flexibility and scalability.
Example Explanation
In the provided example:
- Encapsulation: The Animal class encapsulates attributes (name) and behavior (speak) that define generic animals. Encapsulation ensures that an object’s internal details are hidden, promoting data integrity and modularity.
class Animal:
def __init__(self, name):
self.name = name
- Inheritance: The Dog class inherits from the Animal class, allowing it to reuse and extend its functionality. Inheritance reduces redundancy and promotes code reusability.
class Dog(Animal):
def speak(self):
return f"{self.name} barks"
- Polymorphism: The speak method is overridden in the Dog class to provide behavior specific to dogs. This showcases polymorphism, where the same method (speak) behaves differently depending on the object calling it.
By creating an instance of Dog (dog = Dog(“Buddy”)), the program demonstrates how specific objects can use generalized functionality while adding unique behaviors. OOP concepts make Python suitable for large-scale applications like web frameworks, game development, and machine learning models.
Computer Science Thinking: Key Concepts Explained
Python enables developers to incorporate fundamental computer science principles, such as recursion, searching, sorting, and algorithm efficiency, into their programs. These principles are essential for solving complex problems and optimizing performance.
1. Recursion
Recursion is a technique where a function calls itself to solve smaller instances of the same problem. It’s particularly useful for tasks like calculating factorials, navigating tree structures, or solving puzzles like the Tower of Hanoi. In the factorial example, the function reduces the problem size (n) at each step, reaching the base case (n == 0) where recursion terminates. However, recursive solutions can be computationally expensive for large inputs, which is why understanding recursion depth and memoization is crucial.
def factorial(n):
return 1 if n == 0 else n * factorial(n - 1)
print(factorial(5)) # Output: 120
2. Searching and Sorting
Efficient data handling requires algorithms like binary search for searching and quicksort for sorting. Sorting data is fundamental for search efficiency, as sorted arrays allow faster lookup methods like binary search, which operates in O(log n) time compared to O(n) for linear search.
arr = [4, 2, 9, 1]
sorted_arr = sorted(arr) # Quicksort internally
print(sorted_arr) # Output: [1, 2, 4, 9]
3. Big O Notation
Big O notation evaluates an algorithm’s performance in terms of time and space complexity. For example, traversing a list element by element has a complexity of O(n), while binary search is more efficient at O(log n). Understanding Big O helps in choosing the right algorithms for scalable solutions.
Natural Language Processing (NLP)
Natural Language Processing (NLP) bridges the gap between human language and computers, enabling machines to understand, interpret, and generate text. Python has established itself as a leader in NLP due to its intuitive syntax and robust libraries like NLTK, spaCy, and Transformers, which streamline the implementation of complex linguistic tasks.
For instance, NLTK (Natural Language Toolkit) provides tools for tokenization, stemming, lemmatization, and more, making it ideal for foundational NLP tasks. In the example below, the word_tokenize function breaks a sentence into individual words:
from nltk.tokenize import word_tokenize
text = "Natural language processing is fascinating."
tokens = word_tokenize(text)
print(tokens) # Output: ['Natural', 'language', 'processing', 'is', 'fascinating', '.']
spaCy, on the other hand, excels in processing large text corpora efficiently, offering features like named entity recognition (NER) and dependency parsing. For advanced NLP, libraries like Transformers from Hugging Face enable pre-trained models such as BERT and GPT for sentiment analysis, question answering, and summarization.
Applications of NLP are vast and include:
- Sentiment Analysis: Understanding public sentiment from social media or product reviews.
- Text Summarization: Generating concise summaries of lengthy articles or reports.
- Chatbots: Automating customer support through conversational interfaces.
With these tools, Python empowers professionals to develop solutions ranging from chat assistants to sophisticated language models, making NLP a cornerstone of modern artificial intelligence.
Machine Learning Algorithms: Classification, Regression, and Clustering
Python’s machine learning ecosystem is powered by libraries like Scikit-learn, which simplify the implementation of various algorithms. The three primary types of machine learning tasks—classification, regression, and clustering—are supported with ease.
1. Classification
Classification is a supervised learning task where the goal is to predict categorical labels. For instance, a Decision Tree classifier can predict whether a customer will buy a product (Yes/No) based on features like age and income. In Python, Scikit-learn’s DecisionTreeClassifier is used to train models on labeled data and make predictions. The classifier builds a tree-like structure to decide the class labels based on input features.
from sklearn.tree import DecisionTreeClassifier
X = [[1], [2], [3]]
y = [0, 1, 0]
clf = DecisionTreeClassifier()
clf.fit(X, y)
print(clf.predict([[2]])) # Output: [1]
2. Regression Analysis
Regression is used for predicting continuous outcomes. A simple Linear Regression model can predict numeric values, like house prices, based on features such as square footage. In Scikit-learn, the LinearRegression class helps fit a linear relationship between input features and output labels.
from sklearn.linear_model import LinearRegression
X = [[1], [2], [3]]
y = [2, 4, 6]
model = LinearRegression()
model.fit(X, y)
print(model.predict([[4]])) # Output: [8]
3. Clustering Machine Learning Methode
Clustering is an unsupervised learning method where the goal is to group similar data points together. K-Means clustering divides data into predefined clusters based on feature similarities. Scikit-learn’s KMeans class allows efficient clustering.
from sklearn.cluster import KMeans
X = [[1, 2], [2, 3], [3, 4], [8, 9]]
kmeans = KMeans(n_clusters=2)
kmeans.fit(X)
print(kmeans.labels_) # Output: [0, 0, 0, 1]
These models are foundational tools in Python for various real-world applications.
Deep Learning With Python
Deep learning is a subset of machine learning that uses neural networks with many layers, known as deep neural networks, to solve complex tasks like image recognition, natural language processing (NLP), and speech recognition. Unlike traditional machine learning, which relies on feature extraction, deep learning models automatically learn to identify features from raw data, making them highly effective for tasks where the complexity and volume of data are overwhelming.
Libraries such as TensorFlow and PyTorch have become the go-to tools for building deep learning models. These frameworks simplify the process of designing and training neural networks by providing high-level APIs and pre-built layers for common tasks.
import tensorflow as tf
model = tf.keras.Sequential([
tf.keras.layers.Dense(10, activation='relu'),
tf.keras.layers.Dense(1, activation='sigmoid')
])
model.compile(optimizer='adam', loss='binary_crossentropy')
print("Model built successfully")
In the above code snippet, TensorFlow is used to create a simple feedforward neural network (also known as a fully connected network). The model consists of two layers:
- The first layer has 10 neurons with a ReLU (Rectified Linear Unit) activation function, which helps introduce non-linearity into the model, allowing it to learn complex patterns.
- The second layer is the output layer with 1 neuron and a sigmoid activation function, typically used for binary classification tasks (e.g., spam detection, medical diagnosis).
The model is compiled using the Adam optimizer (which adapts the learning rate) and binary cross-entropy loss (appropriate for binary classification tasks). This deep learning setup provides the foundation for more complex architectures in computer vision, NLP, and beyond.
Final Thoughts
Python is an indispensable tool for modern-day computer scientists and data scientists. From simple scripting to advanced machine learning and NLP, Python offers something for everyone. Its approachable syntax, vast libraries, and applications in AI and deep learning make it a cornerstone of technological innovation.